Learning OpenCV 3. Computer Vision in C++ with the OpenCV Library Adrian Kaehler, Gary Bradski

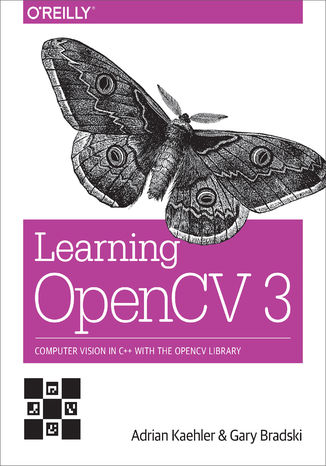
- Autorzy:
- Adrian Kaehler, Gary Bradski
- Wydawnictwo:
- O'Reilly Media
- Ocena:
- Stron:
- 1024
- Dostępne formaty:
-
ePubMobi
Opis
książki
:
Learning OpenCV 3. Computer Vision in C++ with the OpenCV Library
Get started in the rapidly expanding field of computer vision with this practical guide. Written by Adrian Kaehler and Gary Bradski, creator of the open source OpenCV library, this book provides a thorough introduction for developers, academics, roboticists, and hobbyists. You’ll learn what it takes to build applications that enable computers to "see" and make decisions based on that data.
With over 500 functions that span many areas in vision, OpenCV is used for commercial applications such as security, medical imaging, pattern and face recognition, robotics, and factory product inspection. This book gives you a firm grounding in computer vision and OpenCV for building simple or sophisticated vision applications. Hands-on exercises in each chapter help you apply what you’ve learned.
This volume covers the entire library, in its modern C++ implementation, including machine learning tools for computer vision.
- Learn OpenCV data types, array types, and array operations
- Capture and store still and video images with HighGUI
- Transform images to stretch, shrink, warp, remap, and repair
- Explore pattern recognition, including face detection
- Track objects and motion through the visual field
- Reconstruct 3D images from stereo vision
- Discover basic and advanced machine learning techniques in OpenCV
Wybrane bestsellery
O'Reilly Media - inne książki
Dzięki opcji "Druk na żądanie" do sprzedaży wracają tytuły Grupy Helion, które cieszyły sie dużym zainteresowaniem, a których nakład został wyprzedany.
Dla naszych Czytelników wydrukowaliśmy dodatkową pulę egzemplarzy w technice druku cyfrowego.
Co powinieneś wiedzieć o usłudze "Druk na żądanie":
- usługa obejmuje tylko widoczną poniżej listę tytułów, którą na bieżąco aktualizujemy;
- cena książki może być wyższa od początkowej ceny detalicznej, co jest spowodowane kosztami druku cyfrowego (wyższymi niż koszty tradycyjnego druku offsetowego). Obowiązująca cena jest zawsze podawana na stronie WWW książki;
- zawartość książki wraz z dodatkami (płyta CD, DVD) odpowiada jej pierwotnemu wydaniu i jest w pełni komplementarna;
- usługa nie obejmuje książek w kolorze.
Masz pytanie o konkretny tytuł? Napisz do nas: sklep@ebookpoint.pl
Książka drukowana

Oceny i opinie klientów: Learning OpenCV 3. Computer Vision in C++ with the OpenCV Library Adrian Kaehler, Gary Bradski
(0)