Fundamentals of Data Engineering Joe Reis, Matt Housley

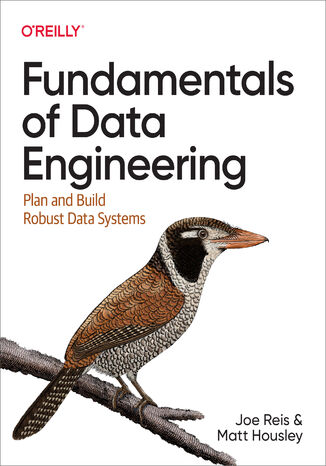
- Autorzy:
- Joe Reis, Matt Housley
- Wydawnictwo:
- O'Reilly Media
- Ocena:
- Stron:
- 450
- Dostępne formaty:
-
ePubMobi
Opis
książki
:
Fundamentals of Data Engineering
Data engineering has grown rapidly in the past decade, leaving many software engineers, data scientists, and analysts looking for a comprehensive view of this practice. With this practical book, you'll learn how to plan and build systems to serve the needs of your organization and customers by evaluating the best technologies available through the framework of the data engineering lifecycle.
Authors Joe Reis and Matt Housley walk you through the data engineering lifecycle and show you how to stitch together a variety of cloud technologies to serve the needs of downstream data consumers. You'll understand how to apply the concepts of data generation, ingestion, orchestration, transformation, storage, and governance that are critical in any data environment regardless of the underlying technology.
This book will help you:
- Get a concise overview of the entire data engineering landscape
- Assess data engineering problems using an end-to-end framework of best practices
- Cut through marketing hype when choosing data technologies, architecture, and processes
- Use the data engineering lifecycle to design and build a robust architecture
- Incorporate data governance and security across the data engineering lifecycle
Wybrane bestsellery
O'Reilly Media - inne książki
Dzięki opcji "Druk na żądanie" do sprzedaży wracają tytuły Grupy Helion, które cieszyły sie dużym zainteresowaniem, a których nakład został wyprzedany.
Dla naszych Czytelników wydrukowaliśmy dodatkową pulę egzemplarzy w technice druku cyfrowego.
Co powinieneś wiedzieć o usłudze "Druk na żądanie":
- usługa obejmuje tylko widoczną poniżej listę tytułów, którą na bieżąco aktualizujemy;
- cena książki może być wyższa od początkowej ceny detalicznej, co jest spowodowane kosztami druku cyfrowego (wyższymi niż koszty tradycyjnego druku offsetowego). Obowiązująca cena jest zawsze podawana na stronie WWW książki;
- zawartość książki wraz z dodatkami (płyta CD, DVD) odpowiada jej pierwotnemu wydaniu i jest w pełni komplementarna;
- usługa nie obejmuje książek w kolorze.
Masz pytanie o konkretny tytuł? Napisz do nas: sklep@ebookpoint.pl
Książka drukowana

Oceny i opinie klientów: Fundamentals of Data Engineering Joe Reis, Matt Housley
(1)(0)
(1)
(0)
(0)
(0)
(0)