Hands-On Gradient Boosting with XGBoost and scikit-learn. Perform accessible machine learning and extreme gradient boosting with Python Corey Wade, Kevin Glynn

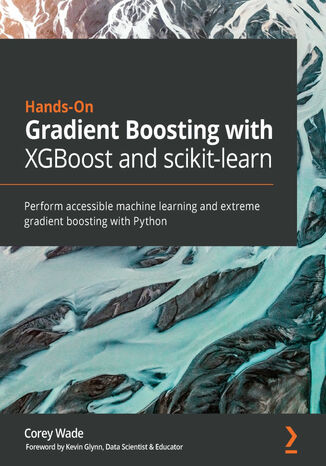
- Autorzy:
- Corey Wade, Kevin Glynn
- Wydawnictwo:
- Packt Publishing
- Ocena:
- Stron:
- 310
- Dostępne formaty:
-
PDFePubMobi
Opis
książki
:
Hands-On Gradient Boosting with XGBoost and scikit-learn. Perform accessible machine learning and extreme gradient boosting with Python
The book introduces machine learning and XGBoost in scikit-learn before building up to the theory behind gradient boosting. You’ll cover decision trees and analyze bagging in the machine learning context, learning hyperparameters that extend to XGBoost along the way. You’ll build gradient boosting models from scratch and extend gradient boosting to big data while recognizing speed limitations using timers. Details in XGBoost are explored with a focus on speed enhancements and deriving parameters mathematically. With the help of detailed case studies, you’ll practice building and fine-tuning XGBoost classifiers and regressors using scikit-learn and the original Python API. You'll leverage XGBoost hyperparameters to improve scores, correct missing values, scale imbalanced datasets, and fine-tune alternative base learners. Finally, you'll apply advanced XGBoost techniques like building non-correlated ensembles, stacking models, and preparing models for industry deployment using sparse matrices, customized transformers, and pipelines.
By the end of the book, you’ll be able to build high-performing machine learning models using XGBoost with minimal errors and maximum speed.
Wybrane bestsellery
Packt Publishing - inne książki
Dzięki opcji "Druk na żądanie" do sprzedaży wracają tytuły Grupy Helion, które cieszyły sie dużym zainteresowaniem, a których nakład został wyprzedany.
Dla naszych Czytelników wydrukowaliśmy dodatkową pulę egzemplarzy w technice druku cyfrowego.
Co powinieneś wiedzieć o usłudze "Druk na żądanie":
- usługa obejmuje tylko widoczną poniżej listę tytułów, którą na bieżąco aktualizujemy;
- cena książki może być wyższa od początkowej ceny detalicznej, co jest spowodowane kosztami druku cyfrowego (wyższymi niż koszty tradycyjnego druku offsetowego). Obowiązująca cena jest zawsze podawana na stronie WWW książki;
- zawartość książki wraz z dodatkami (płyta CD, DVD) odpowiada jej pierwotnemu wydaniu i jest w pełni komplementarna;
- usługa nie obejmuje książek w kolorze.
Masz pytanie o konkretny tytuł? Napisz do nas: sklep@ebookpoint.pl
Książka drukowana

Oceny i opinie klientów: Hands-On Gradient Boosting with XGBoost and scikit-learn. Perform accessible machine learning and extreme gradient boosting with Python Corey Wade, Kevin Glynn
(0)