Applied Machine Learning Solutions with Python Siddhanta Bhatta

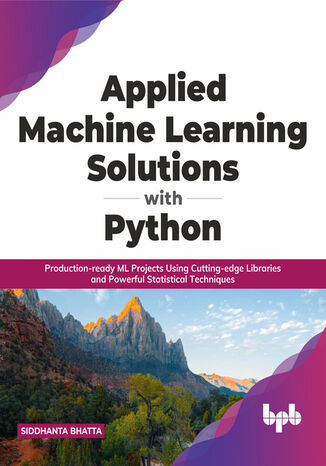
- Autor:
- Siddhanta Bhatta
- Wydawnictwo:
- BPB Publications
- Ocena:
- Stron:
- 356
- Dostępne formaty:
-
ePubMobi
Opis
książki
:
Applied Machine Learning Solutions with Python
Popular techniques for problem formulation, data collection, and data cleaning in machine learning.
Comprehensive and useful machine learning tools such as MLFlow, Streamlit, and many more.
Covers numerous machine learning libraries, including Tensorflow, FastAI, Scikit-Learn, Pandas, and Numpy. Description
This book discusses how to apply machine learning to real-world problems by utilizing real-world data. In this book, you will investigate data sources, become acquainted with data pipelines, and practice how machine learning works through numerous examples and case studies.
The book begins with high-level concepts and implementation (with code!) and progresses towards the real-world of ML systems. It briefly discusses various concepts of Statistics and Linear Algebra. You will learn how to formulate a problem, collect data, build a model, and tune it. You will learn about use cases for data analytics, computer vision, and natural language processing. You will also explore nonlinear architecture, thus enabling you to build models with multiple inputs and outputs. You will get trained on creating a machine learning profile, various machine learning libraries, Statistics, and FAST API.
Throughout the book, you will use Python to experiment with machine learning libraries such as Tensorflow, Scikit-learn, Spacy, and FastAI. The book will help train our models on both Kaggle and our datasets. What you will learn
Construct a machine learning problem, evaluate the feasibility, and gather and clean data.
Learn to explore data first, select, and train machine learning models.
Fine-tune the chosen model, deploy, and monitor it in production.
Discover popular models for data analytics, computer vision, and Natural Language Processing. Who this book is for
This book caters to beginners in machine learning, software engineers, and students who want to gain a good understanding of machine learning concepts and create production-ready ML systems. This book assumes you have a beginner-level understanding of Python. Table of Contents
1. Introduction to Machine Learning
2. Problem Formulation in Machine Learning
3. Data Acquisition and Cleaning
4. Exploratory Data Analysis
5. Model Building and Tuning
6. Taking Our Model into Production
7. Data Analytics Use Case
8. Building a Custom Image Classifier from Scratch
9. Building a News Summarization App Using Transformers
10. Multiple Inputs and Multiple Output Models
11. Contributing to the Community
12. Creating Your Project
13. Crash Course in Numpy, Matplotlib, and Pandas
14. Crash Course in Linear Algebra and Statistics
15. Crash Course in FastAPI About the Authors
Siddhanta Bhatta is a Machine Learning engineer with 6 years of experience in building machine learning products. He is currently working as a Senior Software Engineer in Data Analytics, Machine Learning, and Deep Learning. He has built multiple data apps in various domains such as vision, NLP, Data Analytics, and many more. He is a Microsoft-certified data scientist who believes in data literacy.
LinkedIn Profile: https://www.linkedin.com/in/siddhanta-bhatta-377880a7/
Blog Link: https://joyofunderstanding926957091.wordpress.com/
Wybrane bestsellery
BPB Publications - inne książki
Dzięki opcji "Druk na żądanie" do sprzedaży wracają tytuły Grupy Helion, które cieszyły sie dużym zainteresowaniem, a których nakład został wyprzedany.
Dla naszych Czytelników wydrukowaliśmy dodatkową pulę egzemplarzy w technice druku cyfrowego.
Co powinieneś wiedzieć o usłudze "Druk na żądanie":
- usługa obejmuje tylko widoczną poniżej listę tytułów, którą na bieżąco aktualizujemy;
- cena książki może być wyższa od początkowej ceny detalicznej, co jest spowodowane kosztami druku cyfrowego (wyższymi niż koszty tradycyjnego druku offsetowego). Obowiązująca cena jest zawsze podawana na stronie WWW książki;
- zawartość książki wraz z dodatkami (płyta CD, DVD) odpowiada jej pierwotnemu wydaniu i jest w pełni komplementarna;
- usługa nie obejmuje książek w kolorze.
Masz pytanie o konkretny tytuł? Napisz do nas: sklep@ebookpoint.pl
Książka drukowana

Oceny i opinie klientów: Applied Machine Learning Solutions with Python Siddhanta Bhatta (0)
Weryfikacja opinii następuje na podstawie historii zamowień na koncie Użytkownika umiejszczającego opinię.
Użytkownik mógł otrzymać punkty za opublikowanie opinii uprawniające do uzyskania rabatu w ramach Programu Punktowego.