Quantum Machine Learning and Optimisation in Finance. Drive financial innovation with quantum-powered algorithms and optimisation strategies - Second Edition Antoine Jacquier, Oleksiy Kondratyev, Alexander Lipton, Marcos López de Prado

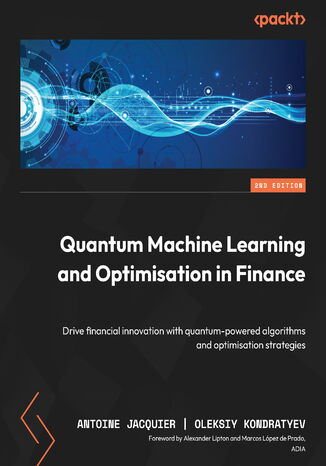
- Autorzy:
- Antoine Jacquier, Oleksiy Kondratyev, Alexander Lipton, Marcos López de Prado
- Serie wydawnicze:
- Hands-on
- Wydawnictwo:
- Packt Publishing
- Ocena:
- Stron:
- 494
- Dostępne formaty:
-
PDFePub
Opis
książki
:
Quantum Machine Learning and Optimisation in Finance. Drive financial innovation with quantum-powered algorithms and optimisation strategies - Second Edition
You’ll get to grips with major QML algorithms that have been widely studied for their transformative potential in finance and learn hybrid quantum-classical computational protocols, the most effective way to leverage quantum and classical computing systems together.
The authors, Antoine Jacquier, a distinguished researcher in quantum computing and stochastic analysis, and Oleksiy Kondratyev, a Quant of the Year awardee with over 20 years in quantitative finance, offer a hardware-agnostic perspective. They present a balanced view of both analog and digital quantum computers, delving into the fundamental characteristics of the algorithms while highlighting the practical limitations of today’s quantum hardware.
By the end of this quantum book, you’ll have a deeper understanding of the significance of quantum computing in finance and the skills needed to apply QML to solve complex challenges, driving innovation in your work.
Wybrane bestsellery
Zobacz pozostałe książki z serii Hands-on
Packt Publishing - inne książki
Dzięki opcji "Druk na żądanie" do sprzedaży wracają tytuły Grupy Helion, które cieszyły sie dużym zainteresowaniem, a których nakład został wyprzedany.
Dla naszych Czytelników wydrukowaliśmy dodatkową pulę egzemplarzy w technice druku cyfrowego.
Co powinieneś wiedzieć o usłudze "Druk na żądanie":
- usługa obejmuje tylko widoczną poniżej listę tytułów, którą na bieżąco aktualizujemy;
- cena książki może być wyższa od początkowej ceny detalicznej, co jest spowodowane kosztami druku cyfrowego (wyższymi niż koszty tradycyjnego druku offsetowego). Obowiązująca cena jest zawsze podawana na stronie WWW książki;
- zawartość książki wraz z dodatkami (płyta CD, DVD) odpowiada jej pierwotnemu wydaniu i jest w pełni komplementarna;
- usługa nie obejmuje książek w kolorze.
Masz pytanie o konkretny tytuł? Napisz do nas: sklep@ebookpoint.pl
Książka drukowana

Oceny i opinie klientów: Quantum Machine Learning and Optimisation in Finance. Drive financial innovation with quantum-powered algorithms and optimisation strategies - Second Edition Antoine Jacquier, Oleksiy Kondratyev, Alexander Lipton, Marcos López de Prado
(0)