
Python Deep Learning. Exploring deep learning techniques and neural network architectures with PyTorch, Keras, and TensorFlow - Second Edition Ivan Vasilev, Daniel Slater, Gianmario Spacagna, Peter Roelants, Valentino Zocca

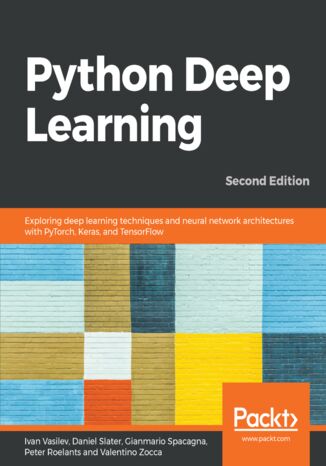
- Autorzy:
- Ivan Vasilev, Daniel Slater, Gianmario Spacagna, Peter Roelants, Valentino Zocca
- Wydawnictwo:
- Packt Publishing
- Ocena:
- Stron:
- 386
- Dostępne formaty:
-
PDFePubMobi

Opis
książki
:
Python Deep Learning. Exploring deep learning techniques and neural network architectures with PyTorch, Keras, and TensorFlow - Second Edition
With the surge in artificial intelligence in applications catering to both business and consumer needs, deep learning is more important than ever for meeting current and future market demands. With this book, you’ll explore deep learning, and learn how to put machine learning to use in your projects.
This second edition of Python Deep Learning will get you up to speed with deep learning, deep neural networks, and how to train them with high-performance algorithms and popular Python frameworks. You’ll uncover different neural network architectures, such as convolutional networks, recurrent neural networks, long short-term memory (LSTM) networks, and capsule networks. You’ll also learn how to solve problems in the fields of computer vision, natural language processing (NLP), and speech recognition. You'll study generative model approaches such as variational autoencoders and Generative Adversarial Networks (GANs) to generate images. As you delve into newly evolved areas of reinforcement learning, you’ll gain an understanding of state-of-the-art algorithms that are the main components behind popular games Go, Atari, and Dota.
By the end of the book, you will be well-versed with the theory of deep learning along with its real-world applications.
This second edition of Python Deep Learning will get you up to speed with deep learning, deep neural networks, and how to train them with high-performance algorithms and popular Python frameworks. You’ll uncover different neural network architectures, such as convolutional networks, recurrent neural networks, long short-term memory (LSTM) networks, and capsule networks. You’ll also learn how to solve problems in the fields of computer vision, natural language processing (NLP), and speech recognition. You'll study generative model approaches such as variational autoencoders and Generative Adversarial Networks (GANs) to generate images. As you delve into newly evolved areas of reinforcement learning, you’ll gain an understanding of state-of-the-art algorithms that are the main components behind popular games Go, Atari, and Dota.
By the end of the book, you will be well-versed with the theory of deep learning along with its real-world applications.
Wybrane bestsellery
Ivan Vasilev, Daniel Slater, Gianmario Spacagna, Peter Roelants, Valentino Zocca - pozostałe książki
Packt Publishing - inne książki
Dzięki opcji "Druk na żądanie" do sprzedaży wracają tytuły Grupy Helion, które cieszyły sie dużym zainteresowaniem, a których nakład został wyprzedany.
Dla naszych Czytelników wydrukowaliśmy dodatkową pulę egzemplarzy w technice druku cyfrowego.
Co powinieneś wiedzieć o usłudze "Druk na żądanie":
- usługa obejmuje tylko widoczną poniżej listę tytułów, którą na bieżąco aktualizujemy;
- cena książki może być wyższa od początkowej ceny detalicznej, co jest spowodowane kosztami druku cyfrowego (wyższymi niż koszty tradycyjnego druku offsetowego). Obowiązująca cena jest zawsze podawana na stronie WWW książki;
- zawartość książki wraz z dodatkami (płyta CD, DVD) odpowiada jej pierwotnemu wydaniu i jest w pełni komplementarna;
- usługa nie obejmuje książek w kolorze.
Masz pytanie o konkretny tytuł? Napisz do nas: sklep@ebookpoint.pl
Proszę wybrać ocenę!
Proszę wpisać opinię!
Książka drukowana

Oceny i opinie klientów: Python Deep Learning. Exploring deep learning techniques and neural network architectures with PyTorch, Keras, and TensorFlow - Second Edition Ivan Vasilev, Daniel Slater, Gianmario Spacagna, Peter Roelants, Valentino Zocca
(0)