Practical Deep Learning at Scale with MLflow. Bridge the gap between offline experimentation and online production Yong Liu, Dr. Matei Zaharia

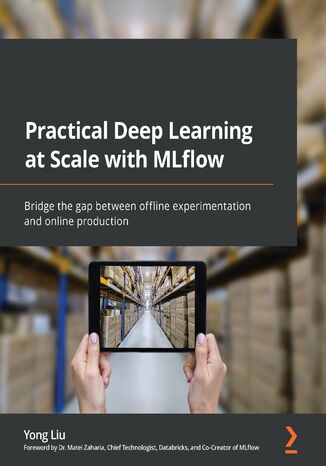
- Autorzy:
- Yong Liu, Dr. Matei Zaharia
- Wydawnictwo:
- Packt Publishing
- Ocena:
- Stron:
- 288
- Dostępne formaty:
-
PDFePub
Opis
książki
:
Practical Deep Learning at Scale with MLflow. Bridge the gap between offline experimentation and online production
From there onward, it guides you step by step in understanding the concept of MLflow experiments and usage patterns, using MLflow as a unified framework to track DL data, code and pipelines, models, parameters, and metrics at scale. You’ll also tackle running DL pipelines in a distributed execution environment with reproducibility and provenance tracking, and tuning DL models through hyperparameter optimization (HPO) with Ray Tune, Optuna, and HyperBand. As you progress, you’ll learn how to build a multi-step DL inference pipeline with preprocessing and postprocessing steps, deploy a DL inference pipeline for production using Ray Serve and AWS SageMaker, and finally create a DL explanation as a service (EaaS) using the popular Shapley Additive Explanations (SHAP) toolbox.
By the end of this book, you’ll have built the foundation and gained the hands-on experience you need to develop a DL pipeline solution from initial offline experimentation to final deployment and production, all within a reproducible and open source framework.
Wybrane bestsellery
Packt Publishing - inne książki
Dzięki opcji "Druk na żądanie" do sprzedaży wracają tytuły Grupy Helion, które cieszyły sie dużym zainteresowaniem, a których nakład został wyprzedany.
Dla naszych Czytelników wydrukowaliśmy dodatkową pulę egzemplarzy w technice druku cyfrowego.
Co powinieneś wiedzieć o usłudze "Druk na żądanie":
- usługa obejmuje tylko widoczną poniżej listę tytułów, którą na bieżąco aktualizujemy;
- cena książki może być wyższa od początkowej ceny detalicznej, co jest spowodowane kosztami druku cyfrowego (wyższymi niż koszty tradycyjnego druku offsetowego). Obowiązująca cena jest zawsze podawana na stronie WWW książki;
- zawartość książki wraz z dodatkami (płyta CD, DVD) odpowiada jej pierwotnemu wydaniu i jest w pełni komplementarna;
- usługa nie obejmuje książek w kolorze.
Masz pytanie o konkretny tytuł? Napisz do nas: sklep@ebookpoint.pl
Książka drukowana

Oceny i opinie klientów: Practical Deep Learning at Scale with MLflow. Bridge the gap between offline experimentation and online production Yong Liu, Dr. Matei Zaharia
(0)