Hands-On Generative Adversarial Networks with PyTorch 1.x. Implement next-generation neural networks to build powerful GAN models using Python John Hany, Greg Walters

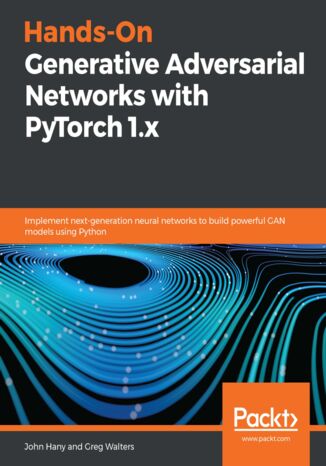
- Autorzy:
- John Hany, Greg Walters
- Wydawnictwo:
- Packt Publishing
- Ocena:
- Stron:
- 312
- Dostępne formaty:
-
PDFePubMobi
Opis
książki
:
Hands-On Generative Adversarial Networks with PyTorch 1.x. Implement next-generation neural networks to build powerful GAN models using Python
This book starts by taking you through the core concepts necessary to understand how each component of a GAN model works. You'll build your first GAN model to understand how generator and discriminator networks function. As you advance, you'll delve into a range of examples and datasets to build a variety of GAN networks using PyTorch functionalities and services, and become well-versed with architectures, training strategies, and evaluation methods for image generation, translation, and restoration. You'll even learn how to apply GAN models to solve problems in areas such as computer vision, multimedia, 3D models, and natural language processing (NLP). The book covers how to overcome the challenges faced while building generative models from scratch. Finally, you'll also discover how to train your GAN models to generate adversarial examples to attack other CNN and GAN models.
By the end of this book, you will have learned how to build, train, and optimize next-generation GAN models and use them to solve a variety of real-world problems.
Wybrane bestsellery
Packt Publishing - inne książki
Dzięki opcji "Druk na żądanie" do sprzedaży wracają tytuły Grupy Helion, które cieszyły sie dużym zainteresowaniem, a których nakład został wyprzedany.
Dla naszych Czytelników wydrukowaliśmy dodatkową pulę egzemplarzy w technice druku cyfrowego.
Co powinieneś wiedzieć o usłudze "Druk na żądanie":
- usługa obejmuje tylko widoczną poniżej listę tytułów, którą na bieżąco aktualizujemy;
- cena książki może być wyższa od początkowej ceny detalicznej, co jest spowodowane kosztami druku cyfrowego (wyższymi niż koszty tradycyjnego druku offsetowego). Obowiązująca cena jest zawsze podawana na stronie WWW książki;
- zawartość książki wraz z dodatkami (płyta CD, DVD) odpowiada jej pierwotnemu wydaniu i jest w pełni komplementarna;
- usługa nie obejmuje książek w kolorze.
Masz pytanie o konkretny tytuł? Napisz do nas: sklep@ebookpoint.pl
Książka drukowana

Oceny i opinie klientów: Hands-On Generative Adversarial Networks with PyTorch 1.x. Implement next-generation neural networks to build powerful GAN models using Python John Hany, Greg Walters (0)
Weryfikacja opinii następuje na podstawie historii zamowień na koncie Użytkownika umiejszczającego opinię.
Użytkownik mógł otrzymać punkty za opublikowanie opinii uprawniające do uzyskania rabatu w ramach Programu Punktowego.