Causal Inference with Bayesian Networks. Exploring the Practical Applications and Demonstrations of Causal Inference using R and Python Yousri El Fattah

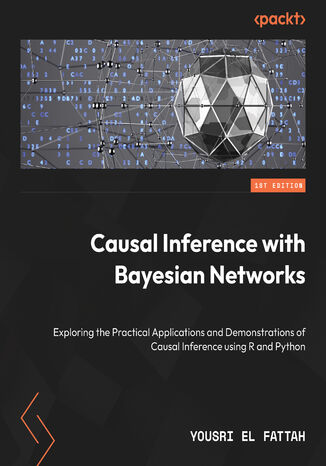
- Autor:
- Yousri El Fattah
- Serie wydawnicze:
- Hands-on
- Wydawnictwo:
- Packt Publishing
- Ocena:
Opis
książki
:
Causal Inference with Bayesian Networks. Exploring the Practical Applications and Demonstrations of Causal Inference using R and Python
For probabilistic inference in Bayesian networks, you will learn methods of variable elimination and tree clustering. For causal inference you will learn the computational framework of Pearl's do-calculus for the identification and estimation of causal effects with causal models. In the context of causal inference with observational data, you will be introduced to the potential outcomes framework and explore various classes of meta-learning algorithms that are used to estimate the conditional average treatment effect in causal inference.
The book includes practical exercises using R and Python for you to engage in and solidify your understanding of different approaches to probabilistic and causal inference. By the end of this book, you will be able to build and deploy your own causal inference application. You will learn from causal inference sample use cases for diagnosis, epidemiology, social sciences, economics, and finance.
Wybrane bestsellery
Zobacz pozostałe książki z serii Hands-on
Packt Publishing - inne książki
Dzięki opcji "Druk na żądanie" do sprzedaży wracają tytuły Grupy Helion, które cieszyły sie dużym zainteresowaniem, a których nakład został wyprzedany.
Dla naszych Czytelników wydrukowaliśmy dodatkową pulę egzemplarzy w technice druku cyfrowego.
Co powinieneś wiedzieć o usłudze "Druk na żądanie":
- usługa obejmuje tylko widoczną poniżej listę tytułów, którą na bieżąco aktualizujemy;
- cena książki może być wyższa od początkowej ceny detalicznej, co jest spowodowane kosztami druku cyfrowego (wyższymi niż koszty tradycyjnego druku offsetowego). Obowiązująca cena jest zawsze podawana na stronie WWW książki;
- zawartość książki wraz z dodatkami (płyta CD, DVD) odpowiada jej pierwotnemu wydaniu i jest w pełni komplementarna;
- usługa nie obejmuje książek w kolorze.
Masz pytanie o konkretny tytuł? Napisz do nas: sklep@ebookpoint.pl
Książka drukowana

Oceny i opinie klientów: Causal Inference with Bayesian Networks. Exploring the Practical Applications and Demonstrations of Causal Inference using R and Python Yousri El Fattah
(0)